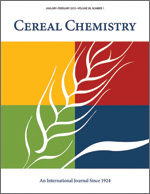
Cereal Chem. 73 (3):399-405 |
VIEW ARTICLE
Analytical Techniques and Instrumentation
Classification of Wheat by Visible and Near-Infrared Reflectance from Single Kernels.
Stephen R. Delwiche (1,2) and David R. Massie (1). Beltsville Agricultural Research Center, Instrumentation and Sensing Laboratory, ARS, USDA, Beltsville, MD 20705. Mention of company or trade names is for purposes of description only and does not imply endorsement by the U.S. Department of Agriculture. Corresponding author. E-mail: <sdelwiche@asrr.arsusda.gov> Accepted January 18, 1996. This article is in the public domain and not copyrightable. It may be freely reprinted with customary crediting of the source. American Association of Cereal Chemists, Inc., 1996.
Identification of wheat class is a necessary component of the official inspection of U.S. wheat, owing to differences in functionality and hence in trade value. Because of the numerous cultivars for the several U.S. wheat classes, segregation by cultivar is generally impractical during postharvest handling. Cultivars of differing wheat classes are sometimes inadvertently mixed, resulting in classification of the lot to a mixed category, thus lowering its value. Single-kernel near-infrared reflectance scans from two spectral regions (551-750 nm for distinctions based on color, 1,120-2,476 nm for distinctions based on intrinsic properties) were collected on 10 randomly drawn kernels from each of 318 unique samples obtained from commercial sources. Partial least squares and multiple linear regression analyses were used to develop binary decision models for various combinations of two wheat classes, choosing from five classes: hard white (HWH), hard red spring (HRS), hard red winter (HRW), soft red winter (SRW), and soft white (SWH). Two-class model accuracy, defined as the proportion of correctly identified kernels of a known wheat class, was greatest (99%) when red and white classes such as HRW vs. HWH were compared. Accuracies declined to typically 78-91% when the two classes were of similar color (e.g., HRW vs. SRW, HWH vs. SWH). Using a cascade of binary comparisons similar to two-class models, a five-class model structure was developed. Five-class model accuracy ranged from 65% for SRW wheat to 92% for SWH.